Predictive maintenance: One of the industrial IoT’s big draws
By TheWAY - 3월 16, 2018
Predictive maintenance: One of the industrial IoT’s big draws
The number-one value-add of IIoT to operation technology so far has been predictive maintenance. Combining machine learning and AI with the data generated by the flood of new IoT devices lets you better understand how systems work and can be maintained.
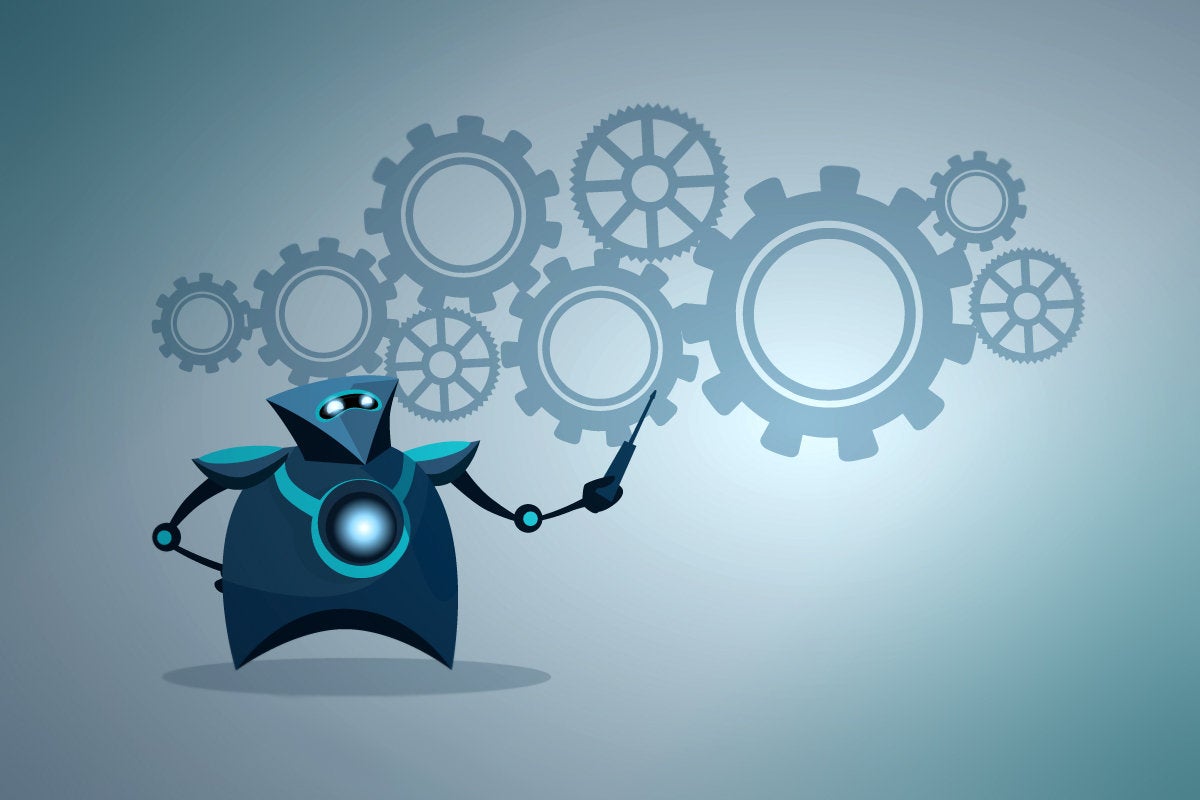
Combining machine learning and artificial intelligence (AI) with the deep pool of data generated by the flood of newly connected devices offers the opportunity to more deeply understand the way complex systems work and interact with each other.
And that can promote predictive maintenance - with the ability to pinoint when components of industrial equipment are likely to fail so they can be replaced or repaired before they do, thereby avoiding more costly damage and downtime.
Fine tuning IIoT predictive maintenance models
According to Wael Elrifai, senior director of sales engineering and data science at Hitachi Vantara – the company’s IoT arm – one of the complexities of predictive maintenance is that AI-produced models for system behavior have to change over time. He used the example of a Hitachi Vantara railway customer with a 27½-year maintenance contract to illustrate the issue.
As train parts age, they respond to stresses differently than they do when they’re new. Because of that, maintenance schedules should be adjusted over time to take into consideration changing failure rates. These schedules can be generated with models that are the output of machine learning, he says.
There's a "bathtub curve" to equipment failure, Elrifai said. At the beginning of its service life, there are frequent failures, but maintenance processes get figured out as time passes, so failures become much rarer. "And then, of course, end-of-life – it starts to fail a lot again," said Elrifai.
This type of AI-produced model can be created for other industries as well, and Hitachi has just released a platform called Lumeda that pulls in IIoT data that data scientists can use to adjust their machine-learning models more precisely. “It’s all about being able to monitor machine-learning-model accuracy after a model goes into production,” said Arik Pelkey, senior director of product marketing.
One example is a chemical-manufacturing process. Lumada creates a centralized data pool on which data scientists can experiment, so the process of testing different models against each other means that the company can change its inputs and get a more accurate prediction of what's going to happen to the chemicals at the other end of the production line.
Elrifai and Pelkey said that the biggest impact that evolving machine-learning-model management will have will be on low-margin, high-capital businesses, like heavy industry and transportation.
IIoT preventive maintenance in cars
Cars manufactured in the past 15 years generally have a computer on board called OBD-II, which stands for on-board diagnostic, version 2. If you’ve seen a mechanic plug a scanner into a specialized port on your car, they’re probably checking with the OBD-II.
A startup called TheCarForce is looking to leverage the data from that computer to help drivers and garages – and ultimately, even manufacturers – alike. CarForce’s hardware is a dongle that plugs into that port and stays there, sending diagnostic data, via a SIM card, back to a central hub.
CarForce founder Jessika Lora said that modern cars are collecting more self-diagnosis data than the space shuttle. But once gathered, the data doesn’t get stored and used for analytics. “It went to the car’s computer and it got discarded immediately," she said.
CarForce is mostly focused on selling its product to garages, but Lora said that the potential beneficiaries are numerous. In the garage use case, mechanics can get real-time maintenance data from vehicles they service, which offers both the ability to warn customers of impending problems and to correlate large data sets together to help predict future reliability issues.
It's a value-add because the garage can stay a step ahead of mechanical issues – an alert goes off, and the garage can contact the customer to schedule maintenance. Even an awareness that customer X might be coming in for an oil change on a given day can help with planning and scheduling.
“If you look at the big data/AI path, step one is just seeing the data,” said Lora. It’s part of what she refers to as the “lilypad” approach to development – building one system to enable a leap to the next lilypad, and so on.
CarForce plans to operate on a population level - predicting reliability and failures across big swaths of the automotive landscape.
“So we can actually start making recommendations not just to garages, but to the manufacturer of the car as well,” Lora said. “When we see these three faults occur in tandem, it means that thing X is about to happen to your car.”
IoT predictive maintenance and farm equipment
Travis Senter of Senter Farms, works about 20,000 acres of row crops in northeast Arkansas, about 40 miles north of Memphis, in the Mississippi River delta. Cotton, long-grain rice, soybeans, corn and wheat. 23 tractors, three combines, two cotton-pickers and four sprayers all hooked into John Deere’s JDLink agricultural IoT system.
“We need this technology to be able to track and see where things are. And if there’s something going on, we need to make sure we can fix it in a hurry, because you can’t afford downtime,” said Senter. “You’ve got your back against the wall every day with weather, with timing, with planting.”
The busy season lasts from roughly mid-March through late October, and machines have to be fully available throughout that time. Deere analyzes even minor alerts – what Senter says might be considered “nuisance” alerts to the operator on the ground – and uses them to draw patterns and conclusions about reliability and service data.
Deere’s IIoT team performs high-level analysis on the data it gets from connected machines and has helped Senter’s operations materially.
“For example, we had a fan drive on the front of an engine, and it would cause a small vibration. The system would detect it, send an error code, you’d look at it, it looks fine,” he said. “Well, [Deere] looked at this stuff, and they’re constantly getting requests to shut some of these off, because they seem like nuisance codes.”
That vibration, however, turned out to be sign of incipient failure on 10 of the 13 tractors the code showed up on. “They were able to fix it with maybe a $200-$300 fix, instead of a $6,000 fix to replace that entire drive,” Senter said.
Join the Network World communities on Facebook and LinkedIn to comment on topics that are top of mind.
soource: https://www.networkworld.com/article/3262167/internet-of-things/predictive-maintenance-one-of-the-industrial-iots-big-draws.html
0 개의 댓글