Artificial intelligence in cybersecurity- Caleb Fenton answers readers' questions
By TheWAY - 5월 10, 2019
Artificial intelligence in cybersecurity- Caleb Fenton answers readers' questions
Questions answered about how AI detects threats, how to evaluate AI solutions, whether attackers can hide from AI and more.
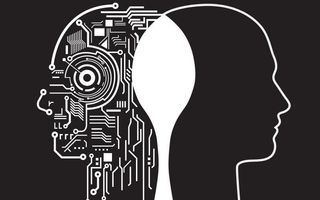
Is AI the Silver Bullet of Cybersecurity?
Two years ago, I talked about how we were in the early stages of the artificial intelligence revolution and how to evaluate AI in security products. Since then, AI research continues to blow minds, particularly with Generative Adversarial Networks (GAN), which are being used to clone voices, generate big chunks of coherent text, and even create creepy pictures of faces of people who don’t exist. With all these cool developments making headlines, it’s no wonder that people want to understand how AI works and how it can be applied to different industries like cyber security.
Unfortunately, there’s no such thing as a silver bullet in security, and you should run away from anyone who says they’re selling one. Security will always be an arms race between attackers and defenders. That being said, AI is an incredibly powerful tool because it allows us to create software which does the job of a malware analyst. It takes a human years of experience and training to develop the skills and intuition to sniff out malware. Now we can train a program to do the same thing in just a few hours with AI learning algorithms and a huge amount of data. To be fair, an analyst is still better, but the gap is closing all the time and AI models only take seconds to analyse a file where a human analyst could take hours or days.
How Does AI Detect Threats?
There are two main approaches for AI-based malware detection on the endpoint right now: looking at files and monitoring behaviours. The former approach uses static features — the actual bytes of the file and information collecting by parsing file structures. Static features are things like PE section count, file entropy, opcode histograms, function imports and exports, and so on. These features are similar to what an analyst might look at to see what a file is capable of.
With enough data, the learning algorithm can generalise or “learn” how to distinguish between good and bad files. This means a well built model can detect malware that wasn’t in the training set. This makes sense because you’re “teaching” software to do the job of a malware analyst. Traditional, signature-based detection, by contrast, generally requires getting a copy of the malware file and creating signatures, which users would then need to download, sometimes several times a day, to be protected.
The other type of AI-based approach is training a model on how programs behave. The real trick here is how you define and capture behaviour. Monitoring behaviour is a tricky, complex problem, and you want to feed your algorithm robust, informative, context-rich data which really captures the essence of a program’s execution. To do this, you need to monitor the operating system at a very low level and, most importantly, link individual behaviours together to create full “storylines”. For example, if a program executes another program, or uses the operating system to schedule itself to execute on boot up, you don’t want to consider these different, isolated executions, but a single story.
Training AI models on behavioral data is similar to training static models, but with the added complexity of the time dimension. In other words, instead of evaluating all features at once, you need to consider cumulative behaviors up to various points in time. Interestingly, if you have good enough data, you don’t really need an AI model to convict an execution as malicious. For example, if the program starts executing but has no user interaction, then it tries to register itself to start when the machine is booted, then it starts listening to keystrokes, you could say it’s very likely a keylogger and should be stopped. These types of expressive “heuristics” are only possible with a robust behavioral engine.
How Do You Evaluate AI Solutions?
This question comes up a lot, and understandably so. I’ve written about this before in What Really Matters with Machine Learning. Essentially, since AI is so new, people don’t know the right questions to ask, and there’s a lot of marketing hype distorting what’s truly important.
The important thing to remember is that AI is essentially teaching a machine, so you shouldn’t really care how it was taught. Instead, you should only care how well it has learned. For example, instead of asking what training algorithm was used (e.g. neural network, SVM, etc), ask how the performance was tested and how well it did. They should probably be using k-fold cross validation to know if they’re overfitting the model and generalising well, and they should optimise for precision to avoid false positives. Of course, raw model performance won’t be an indicator of how well the product works because the model is probably just one component in a suite of detection mechanisms.
Another important consideration is training data quality. Consider for example two people trying to learn advanced calculus. The first person practices by solving 1,000,000 highly similar problems from the first chapter of the book. The second person practices by only solving 100 problems, but made sure that those 100 problems were similar to and more difficult than questions on practice tests. Which person do you think will learn calculus better? Likewise for AI, you shouldn’t bother asking how many features or training samples are used. Instead, ask how data quality is measured and how informative the features are. With machine learning, it’s garbage in, garbage out, and it’s important to ensure training data are highly varied, unbiased, and similar to what’s seen in the wild.
Can Attackers Hide from AI Detection?
Since static and dynamic AI are both very different, adversaries must use totally different evasion techniques for each one. However, it should be noted that since AI is still fairly new, many attackers have not fully adapted and are not actively seeking to evade AI solutions specifically. They still rely heavily on traditional evasion techniques such as packing, obfuscation, droppers & payloads, process injection, and tampering with the detection products directly.
If attackers want to avoid static AI detection, they essentially must change how their compiled binary looks, and since it’s impossible to know how they should change it a priori, they’ll have to try a bunch of variations of source code modification, compilation options, and obfuscation techniques until they find one that isn’t detected. This is a lot of work, and it scales up with the number of products they’re trying to avoid.
We’re just starting to see some interesting research on how to defeat static AI with Machine Learning Poisoning and Adversarial Machine Learning. To quickly summarise how adversarial machine learning works, imagine an image classification AI which can recognise, say, a picture of a panda. If the attacker has access to the AI model, they can “fuzz” it by adding a bit of “noise” to the image so that the model is confused and the image is recognised as something completely different. Here’s a picture illustrating this:
Machine Learning Poisoning and Adversarial Machine Learning.
This type of attacker-focused research is helpful to defence researchers like me because we can understand the attacks we’ll need to defend against before attackers start using them. That being said, these attacks are pretty sophisticated and we haven’t really seen this being used in the wild yet. However, new technology is always adopted where friction is highest. In other words, attackers will pay the “cost” of learning and implementing these new attacks if AI detection becomes enough of a hassle for them. But then we’ll be there to create new defences against those attacks. It’s a never-ending game of cat and mouse.
Avoiding behavioural AI, on the other hand, is much more challenging. Why? Because fundamentally malware must not behave maliciously to avoid detection. This is, understandably, difficult. For example, malware may successfully run and even achieve persistence without being detected, but once running the malware would eventually need to do something malicious like log and exfiltrate personal data, encrypt files and ask for ransom, or spread to more endpoints. A good behavioural detection should monitor for all these types of activities.
Can Hackers Hide Their Tracks?
It depends a lot on how much is being logged. It used to be the case that if you compromised a system, you only had to clear a few log files and it was like you were never there. But modern operating systems are more advanced and there are different logging tools out there, so it’s more difficult to be aware of them all. Additionally, the network itself may be monitored, and networking hardware may not be possible for the attacker to penetrate. Finally, many EDR solutions constantly report endpoint telemetry to the cloud which is inaccessible to attackers.
As security products improve, even if an attack goes unnoticed at first and an attacker tries to cover their trails, it’s increasingly possible to discover the attack and at least partially reconstruct the attack storyline. Since no security product is perfect, I think it’s really important to focus on arming security teams with as much information and context as possible so that they can find missed attacks and understand the full scope of the breach.
Do you have questions about AI in Cyber Security? Contact us at SentinelOne or try a demo and see how it works in practice.
0 개의 댓글